Electronic Health Records Machine Learning
1. introduction. electronic health records (ehr) systems store data associated with each individual’s health journey (including demographic information, diagnoses, medications, laboratory tests and results, medical images, clinical notes, and more). while the primary use of ehr was to improve the efficiency and ease of access of health systems it has found a lot of applications in clinical. Machine learning (ml) is a powerful electronic health records machine learning tool that delivers insights hidden in internet of things (iot) data. these hybrid technologies work smartly to improve the decision-making process in different areas such as education, security, business, and the healthcare industry. ml empowers the iot to demystify hidden patterns in bulk data for optimal prediction and recommendation systems. healthcare.
In this way, machine learning algorithms, play a major role in revolutionizing the growing electronic health records. future scope of machine learning in revolutionizing health data and its services with the data analytics is already put to work electronic health records machine learning as the electronic health records were introduced, the future of the healthcare market lies in the. Predictive modeling with electronic health record (ehr) data is anticipated to drive personalized medicine and improve healthcare quality. in proceedings of the 1st machine learning for. The combination of these two resulted in the adoption of electronic health records (ehr) that use a data science toolkit for the benefit of medical procedures. in addition to this, healthcare is the perfect material for various machine learning algorithms to streamline workflows, modernize database maintenance, and increase the accuracy of results.
The Trimmer Place Officesupplynow Com
Machine Learning To Revolutionize The Growing Electronic
clicks view demo fully customizable templates fully customizable electronic health record templates allow electronic health records machine learning you to efficiently chart while maintaining detailed documentations of your patients’ visits machine learning algorithm veracity remembers preference of treatment and episodes Machine learning, march 2016, volume 102, issue 3, pp 323-348. online first: october 2015. pdf, arxiv [ml] p. schulam, f. wigley, s. saria. clustering longitudinal clinical marker trajectories from electronic health data: applications to phenotyping and endotype discovery. american association for artificial intelligence, january 2015. pdf. Electronichealthrecord–derived data and novel analytics, such as machine learning, offer promising approaches to identify high-risk patients and inform nursing practice. purpose: the aim was to identify patients at risk for readmissions by applying a machine-learning technique, classification and regression tree, to electronic health record. Founded in 2010, new york-based prognos claims that it uses machine learning to run its software which claims to analyze electronic medical records from various hospitals and healthcare systems. the company says that its database of clinical diagnostics information includes data for 50 diseases and its 1,000 algorithms are trained to analyze.
Comparison Of Electronic Records To Paper Records In Mental
What do you get when you juxtapose two of the hottest domains today ai and healthcare? a peek into the future, potentially. Most electronic health records (ehrs) are built on technology that is 20 or 30 years old. generally, ehrs have kept up with rapid changes in healthcare by making incremental improvements over time. but it is becoming more and more challenging to retrofit ehrs to take full advantage of new innovations such as the cloud, artificial.
The wide adoption of electronic health records (ehr) electronic health records machine learning has resulted in large amounts of clinical data becoming available, which promises to support service delivery and advance clinical and informatics research. deep learning techniques have demonstrated performance in predictive analytic tasks using ehrs yet they typically lack model result transparency or explainability functionalities and. 1uphealth provides 100s of health tech companies and systems with the tools they need to create easy and accessible experiences for their users. our ehr connectivity covers millions of patient medical records. the fhir api platform enables teams to build in minutes what would have previously taken years.
Can machine learning, interoperability, and the judy faulkner touch bring joyful usability to electronic health records? source: epic systems september 27, 2017 a public appearance by epic systems founder and ceo judy faulkner is always a big event for the health it industry, and nothing is bigger for epic than the electronic health record. Federal and state governments, insurance companies and other large medical institutions are heavily promoting the adoption of electronic health records. the us congress included a formula of both incentives (up to $44,000 per physician under medicare, or up to $65,000 over six years under medicaid) and penalties (i. e. decreased medicare and medicaid reimbursements to doctors who fail to use.
Electronicmedicalrecords And Machine Learning In
Trends In Using Iot With Machine Learning In Health
This special communication outlines the potential biases that may be introduced into machine learning–based clinical decision support tools that use electronic health record data and proposes potential solutions to the problems of overreliance on automation, algorithms based on biased data, and algorithms that do not provide information that. We will now apply the knowledge gained from the grus tutorial and part 1 of this series to a larger publicly available ehr dataset. this study will utilize the mimic iii electronic health record (ehr) dataset, which is comprised of over 58,000 hospital admissions for 38,645 adults and 7875 neonates.
Predictive modeling of depression and anxiety using electronic health records and a novel machine learning approach with artificial intelligence matthew d. nemesure 1 2 michael v. heinz 1 3. Machine learning is an aspect of artificial intelligence that has been aiding in predictive analysis in many different industries, with healthcare being one of the most utilized thanks to some stellar funding. as the name would suggest, machine learning is the process by which computers recognize trends and analyze the data without human input.
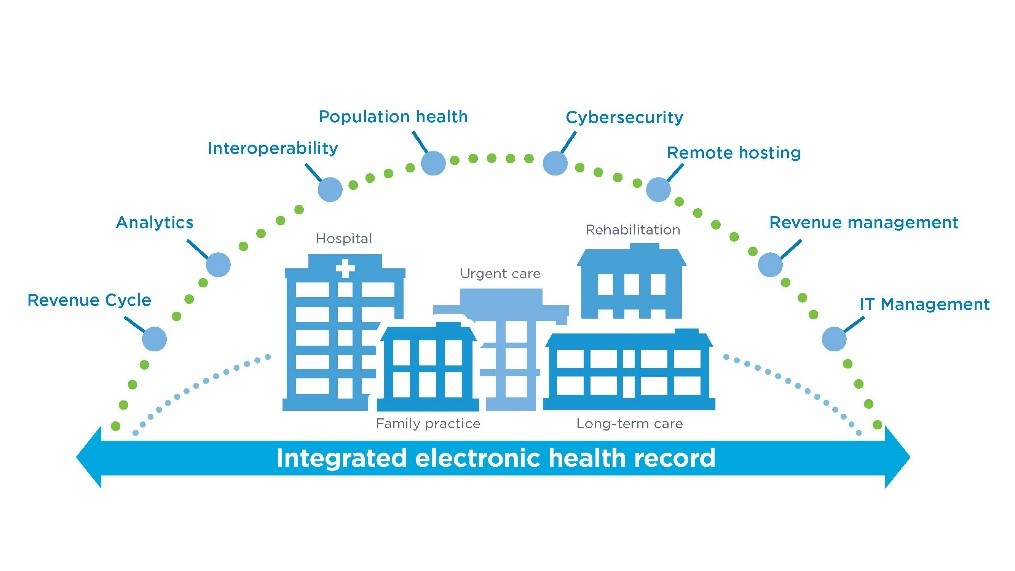
Creating a health care organization that is fully committed to safeguarding personal health information is difficult. it requires managers and employees, both individually and collectively, to engage in an ongoing process of learning, evaluation, and improvement to create an environment—and an organizational culture 3 —that values and respects patients' rights to privacy. Using several machine learning tools, wong et al 1 predicted delirium risk for newly hospitalized patients with high-dimensional electronic health record data at a large academic health institution. they compared these approaches with a questionnaire-based scoring system and found improved performance for machine learning with respect to. Electronicmedicalrecords (emrs) were primarily introduced as a digital health tool in hospitals to improve patient care, but over the past decade, research works have implemented emr data in clinical trials and omics studies to increase translational potential in drug development. emrs could help discover phenotype-genotype associations, enhance clinical trial protocols, automate adverse.
Emar speeds up the prescribing process for patients and physicians. it also complies with federal regulations developed as a result of the american recovery and reinvestment act (arra) of 2009. u. s. laws mandate that all health care facilities and doctors' offices move from paper medical records to electronic medical records (emr). Using electronic health records and machine learning to predict postpartum depression stud health technol inform. 2019 aug 21;264:888-892. doi: 10. 3233/shti190351. Using electronic health records and machine learning to predict postpartum depression. wang s(1)(2), pathak j(1), zhang y(1). author information: (1)weill cornell medicine, cornell university, new york, new york, usa. (2)school of public health, school of medicine, zhejiang university, zhejiang, china. Machinelearning models in electronic health records can outperform conventional survival models for predicting patient mortality in coronary artery disease andrew j. steele roles conceptualization, data curation, formal analysis, investigation, methodology, software, validation, visualization, writing original draft, writing review.

Garg r, dong s, shah s, jonnalagadda sr. a bootstrap machine learning approach to identify rare disease patients from electronic health records. arxiv preprint arxiv:160901586. 2016. 5. colbaugh r, glass k, rudolf c, global mtv. learning to identify rare disease patients from electronic health records. in: amia annual symposium proceedings. How it’s using machine learning in healthcare: with the help of machine learning, quotient health developed software that aims to “reduce the cost of supporting emr [electronic medical records] systems” by optimizing and standardizing the way those systems are designed. the ultimate goal is improved care at a lower cost. This study was a retrospective chart review of medication information compiled before and after the adoption of electronic medical records, comparing archived paper records to recent electronic records for the completeness and retrieval time of documentation at three mental health centers. carrying cases carts & stands cash handling classroom teaching & learning materials cleaning products cleaning tools computer hardware & accessories computer keyboards & mice computer software crafts & recreation room products cups plates & utensils cutting & measuring devices data storage media & supplies desk accessories & workspace organizers electronics envelopes mailers & shipping supplies first aid & health supplies general office accessories hardware tools & accessories identification

Post a Comment for "Electronic Health Records Machine Learning"